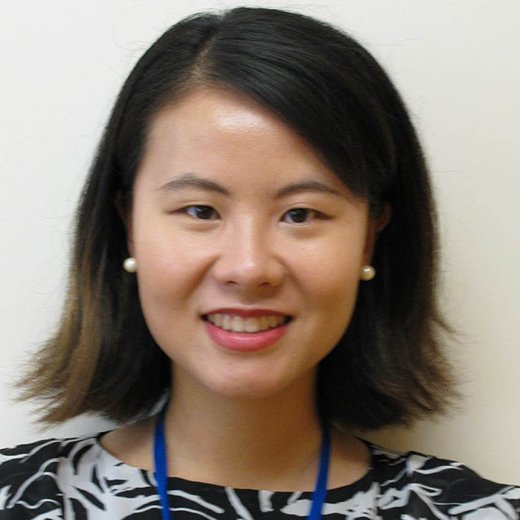
Related
Yun Zhang, PhD, is an assistant professor in the Informatics Department at the J. Craig Venter Institute (JCVI). She received an MMath in Mathematics and Statistics from the University of Oxford, UK, and a PhD in Statistics from the University of Rochester Medical Center. She also has industrial and research experience in Novartis Oncology and Mayo Clinic.
Dr. Zhang’s research interest includes statistical modeling and methodology development for big data produced by advanced biotechnologies. She is experienced in analyzing time-course microarray data, DNA methylation data, and microRNA sequencing data. She is also a professional developer of R and Bioconductor packages. Her recent focus is on applying statistical approaches to single cell RNA sequencing (scRNAseq) data.
Research Priorities
Mapping cell populations in scRNAseq data
- Development of statistical approach for comparing new experimental data with cell type reference definitions to determine if new data represent existing or novel cell types
- Development of statistically-comparable representation of reference cell types for the Human Cell Atlas
Gene set enrichment analysis (GSEA) pipelines with overlapping genes
- Established pipeline FUNNEL-GSEA for time-course gene expression data using functional data analysis techniques
- Development of data-driven method to empirically decompose the gene membership among multiple overlapped pathways
- Extension of FUNNEL to data with limited time points, e.g. cross-sectional data, pre-post study, etc.
Investigation of tissue composition on gene co-expression
- Investigation of the effect of composite cellular types on reconstruction of gene co-expression network
- Application of deconvolution algorithm to tissue composition problems
Publications
Scientific data. 2023-01-24; 10.1: 50.
Brain Data Standards - A method for building data-driven cell-type ontologies
Bioinformatics (Oxford, England). 2022-10-14; 38.20: 4735-4744.
FastMix: a versatile data integration pipeline for cell type-specific biomarker inference
PloS one. 2022-09-23; 17.9: e0275070.
Machine learning for cell type classification from single nucleus RNA sequencing data
Scientific reports. 2022-06-15; 12.1: 9996.
Cell type matching in single-cell RNA-sequencing data using FR-Match
Nature. 2022-04-01; 604.7904: E8.
Author Correction: Comparative cellular analysis of motor cortex in human, marmoset and mouse
Journal of leukocyte biology. 2021-12-01; 110.6: 1225-1239.
Corticosteroid treatment in COVID-19 modulates host inflammatory responses and transcriptional signatures of immune dysregulation
Frontiers in immunology. 2021-10-29; 12.690470.
Machine Learning-Based Single Cell and Integrative Analysis Reveals That Baseline mDC Predisposition Correlates With Hepatitis B Vaccine Antibody Response
Nature. 2021-10-06; 598.7879: 111-119.
Comparative cellular analysis of motor cortex in human, marmoset and mouse
Genome research. 2021-10-01; 31.10: 1767-1780.
A machine learning method for the discovery of minimum marker gene combinations for cell type identification from single-cell RNA sequencing
Briefings in bioinformatics. 2021-07-20; 22.4:
FR-Match: robust matching of cell type clusters from single cell RNA sequencing data using the Friedman-Rafsky non-parametric test
Scientific reports. 2020-05-14; 10.1: 7954.
Longitudinal Study of Oral Microbiome Variation in Twins
Briefings in bioinformatics. 2019-12-08;
The effect of tissue composition on gene co-expression
Frontiers in immunology. 2019-11-12; 10.2602.
Host-Microbial Interactions in Systemic Lupus Erythematosus and Periodontitis
BMC bioinformatics. 2019-04-15; 20.1: 185.
Highly efficient hypothesis testing methods for regression-type tests with correlated observations and heterogeneous variance structure
Briefings in bioinformatics. 2018-05-01; 19.3: 374-386.
Statistical method evaluation for differentially methylated CpGs in base resolution next-generation DNA sequencing data
Bioinformatics (Oxford, England). 2017-07-01; 33.13: 1944-1952.
FUNNEL-GSEA: FUNctioNal ELastic-net regression in time-course gene set enrichment analysis
Research Priorities
Mapping cell populations in scRNAseq data
- Development of statistical approach for comparing new experimental data with cell type reference definitions to determine if new data represent existing or novel cell types
- Development of statistically-comparable representation of reference cell types for the Human Cell Atlas
Gene set enrichment analysis (GSEA) pipelines with overlapping genes
- Established pipeline FUNNEL-GSEA for time-course gene expression data using functional data analysis techniques
- Development of data-driven method to empirically decompose the gene membership among multiple overlapped pathways
- Extension of FUNNEL to data with limited time points, e.g. cross-sectional data, pre-post study, etc.
Investigation of tissue composition on gene co-expression
- Investigation of the effect of composite cellular types on reconstruction of gene co-expression network
- Application of deconvolution algorithm to tissue composition problems