Teaching Computers to Recognize Sick Guts: Machine-Learning and the Microbiome
Published Date
By:
- Tiffany Fox
Share This:
Article Content
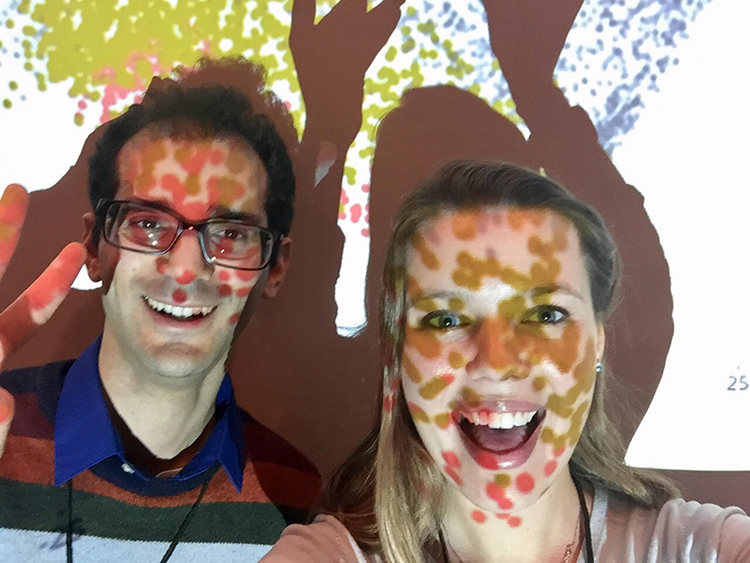
A visualization of protein families is projected onto researchers Mehrdad Yazdani and Bryn Taylor in the UC San Diego Center for Microbiome Innovation. Selfie by Bryn Taylor.
A new proof-of-concept study by researchers from the University of California San Diego succeeded in training computers to “learn” what a healthy versus an unhealthy gut microbiome looks like based on its genetic makeup. Since this can be done by genetically sequencing fecal samples, the research suggests there is great promise for new diagnostic tools that are, unlike blood draws, non-invasive.
As recent advances in scientific understanding of Parkinson’s disease and cancer immunotherapy have shown, our gut microbiomes – the trillions of bacteria, viruses and other microbes that live within us – are emerging as one of the richest untapped sources of insight into human health.
The problem is these microbes live in a very dense ecology of up to 1 billion micobes per gram of stool. Imagine the challenge of trying to specify all the different animals and plants in a complex ecology like a rain forest or coral reef – and then imagine trying to do this in the gut microbiome, where each creature is microscopic and identified by its DNA sequence. Determining the state of that ecology is a classic Big Data problem, where the Big Data is provided by a powerful combination of genetic sequencing techniques and supercomputing software tools. The challenge then becomes how to mine this Big Data to obtain new insights into the causes of diseases, as well as novel therapies to treat them.
The new paper, titled “Using Machine Learning to Identify Major Shifts in Human Gut Microbiome Protein Family Abundance in Disease,” was presented last month at the IEEE International Conference on Big Data. It was written by a joint research team from UC San Diego and the J. Craig Venter Institute (JCVI). At UC San Diego, it included machine learning and data scientist Mehrdad Yazdani at the California Institute for Telecommunications and Information Technology’s (Calit2) Qualcomm Institute, Biomedical Sciences graduate student Bryn C. Taylor and Pediatrics Postdoctoral Scholar Justine Debelius, as well as Rob Knight, professor in the UC San Diego School of Medicine's Pediatrics Department as well as the Computer Science and Engineering Department, and director of the Center for Microbiome Innovation, and Larry Smarr, Director of Calit2 and a professor of Computer Science and Engineering. The UC San Diego team also collaborated with Weizhong Li, an associate professor at JCVI.
The work began with a genetic sequencing technique known as “metagenomics,” which breaks up the DNA of the hundreds of species of microbes that live in the human large intestine (our “gut”). The technique was applied to 30 healthy people (using sequencing data from the National Institutes of Health’s Human Microbiome Program), together with 30 samples from people suffering from the autoimmune Inflammatory Bowel Disease (IBD), including those with ulcerative colitis and with illeal or colonic Crohn’s disease. This resulted in sequencing around 600 billion DNA bases, which were then fed into the supercomputer to reconstruct the relative abundance of these species; for instance, how many E. coli are present compared to other bacterial species.
Since each bacterium’s genome contains thousands of genes and each gene can express a protein, this technique made it possible to translate the reconstructed DNA of the microbial community into hundreds of thousands of proteins, which are then grouped into about 10,000 protein families. The software to carry this out was developed by Li and then run on the Gordon supercomputer at the San Diego Supercomputer Center (SDSC) using 180,000 core-hours (equivalent to running a PC 24 hours a day for 20 years).
To discover the patterns hidden in this huge pile of numbers, the researchers harnessed what they refer to as “fairly out-of-the-bag” machine-learning techniques originally developed for spam filters and other data mining applications. Their goal was to use these algorithms to classify major changes in the protein families found in the gut bacteria of both healthy subjects and those with IBD, based on the DNA found in their fecal samples.
The researchers first used standard biostatistics routines to identify the 100 most statistically significant protein families that differentiate health and disease states. These 100 protein families were then used as a “training set” to build a machine learning classifier that could classify the remaining 9,900 protein families in diseased versus healthy states. The goal was to find a “signature” for which protein families were elevated or suppressed in disease vs. healthy states.
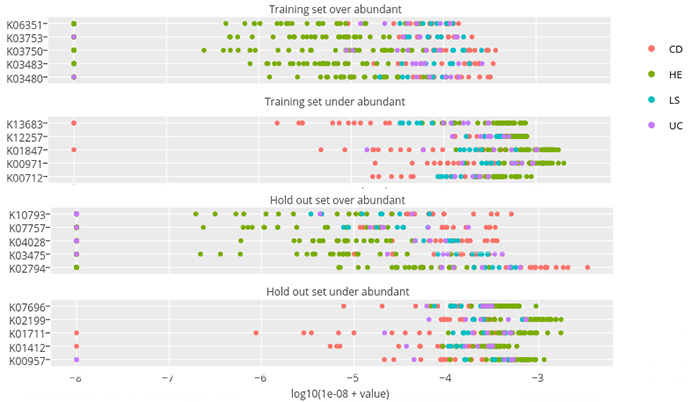
In this figure from the paper, some representative protein families are on the left-hand side of each graph. Each colored dot represents the abundance of that protein family (measured on a logarithmic scale) for a particular fecal sample taken from a healthy person (green dots) or a person with IBD (red, blue, purple dots). Selected for the top two graphs was a “training set” of 100 protein families that statistically differentiated healthy from disease states, which shows that for patients with IBD, certain protein families are either over-abundant (first graph) or under-abundant (second graph) compared with healthy subjects. The lower two graphs are the results from the machine learning algorithm, which discovered the protein families that had similar patterns in the remaining 9,900 protein families. Note that since the scale is logarithmic, these differences in abundance are often 100 to 1 or more.
The process is akin to training a computer to recognize the different flavors of fruit juices – something a human toddler could do intuitively, albeit from a limited perspective.
“From your past experiences drinking juice, you know the difference between orange, apple, and cranberry juice,” Taylor noted. “Your future decision about what juice you are drinking will be based on your past preferences. But it’s really hard to figure out what apple juice tastes like without experiencing it first.”
They have to train the computer, in other words, to recognize what apple juice tastes like – or in this case, what a “healthy” microbiome looks like by clustering data according to bacteria.
“You can try to categorize healthy and sick people by looking at their intestinal bacterial composition,” explained Taylor, “but the differences are not always clear. Instead, when we categorize by the bacterial protein family levels, we see a distinct difference between healthy and sick people. This is because proteins are the workhorses of biology, and by analyzing the proteins produced by these bacteria, we can get an idea of what the bacteria are doing in your gut."
The machine-learning approach is effective, said Yazdani, precisely because it’s statistically based. “The rules are not set in stone,” he added. “What you need is past data and past experiences from patients, and then based on statistics or distribution you make your decisions. You let the data speak for itself.”
Since Smarr suffers from Crohn’s disease, he has been working with Knight’s Center for Microbiome Innovation to advance research in this area. “Because of the exponential increase in the data on your daily changing gut microbiome,” noted Smarr, “it will be essential to develop new machine-learning approaches to bring the biomedically important facets to light.”
In the future, the researchers hope to expand their analysis, using SDSC’s Comet supercomputer, from 10,000 protein families to one million individual genes, each of which codes for a protein which can be expressed in the gut microbiome. “Scalable methods for quickly identifying such anomalies between health and disease states will be increasingly valuable for biological interpretation of sequence data,” they wrote in the paper, which they completed in eight intense days.
“We wanted a fast turn-around,” said Yazdani. “That’s really important, especially for clinical data.”
Following peer review, the paper was one of only 20 percent of the 423 submissions that were accepted as regular papers for the 2016 IEEE International Conference on Big Data. The conference was held in Washington, D.C. on Dec. 5-8, 2016. Yazdani and Taylor attended and presented the paper.
Share This:
You May Also Like
Stay in the Know
Keep up with all the latest from UC San Diego. Subscribe to the newsletter today.